Digital Health: AI Bias & Ethics Resources
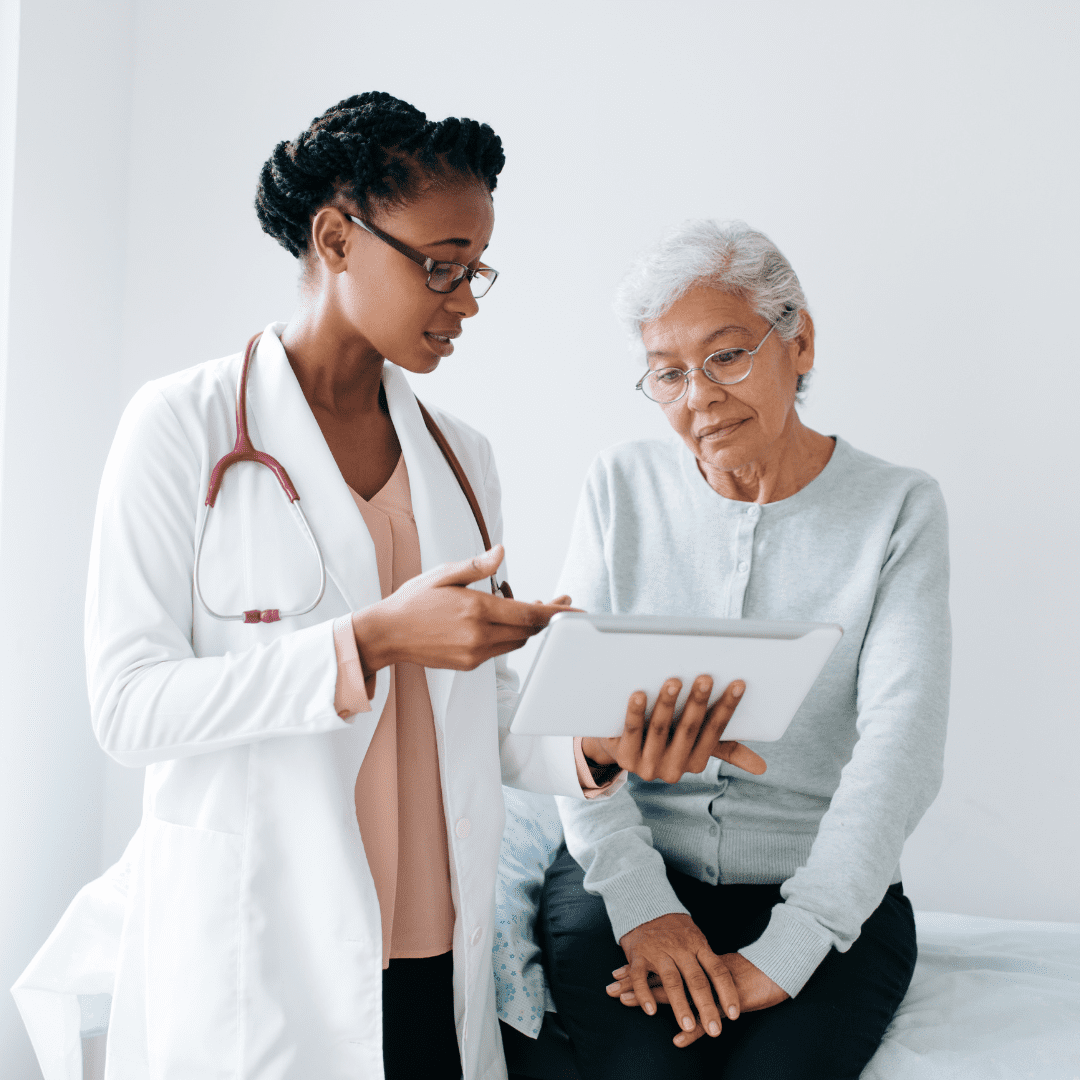
On 6/14, PACT held a Digital Health webinar on AI Bias and Ethics. As a community, attention to the needs of all diverse and intersectional populations is crucial. Below, please find the recording of the webinar and resources from our speakers, who included:
- Stephanie Gervasi, Manager of Data Science, Independence Blue Cross
- Dr. Seun Ross, Executive Director, Health Equity, Independence Blue Cross
- Irene Chen, PhD student – Machine Learning, MIT
- Jaya Aysola, MD, MPH, University of Pennsylvania
- Ryan Wesley Brown, Associate, Duane Morris LLP (Professional Bio)
Resources from the workshop:
Definitions to be shared:
- Artificial Intelligence: The ability of a computer or a robot controlled by a computer to do tasks that are usually done by humans because they require human intelligence and discernment.
- Machine Learning: A type of artificial intelligence (AI) that allows software applications to become more accurate at predicting outcomes without being explicitly programmed to do so. Machine learning algorithms use historical data as input to predict new output values.
- Algorithm: A process or set of rules to be followed in calculations or other problem-solving operations, especially by a computer.
- Algorithmic bias: Describes systematic and repeatable errors in a computer system that create unfair outcomes, such as privileging or benefiting one category over another in ways different from the intended function of the algorithm.
- Fairness: Impartial and just treatment or behavior without favoritism or discrimination. Many types and definitions of fairness exist such as individual, group, and representational fairness.
- Social determinants of health: the conditions in the environments where people are born, live, learn, work, play, worship, and age that affect a wide range of health, functioning, and quality-of-life outcomes and risks.
- HIPAA: The Health Insurance Portability and Accountability Act of 1996 (HIPAA) is a federal law that required the creation of national standards to protect sensitive patient health information from being disclosed without the patient’s consent or knowledge.
References to be shared:
- Sun M, Oliwa T, Peek ME, Tung EL (2022) Negative patient descriptors: documenting racial bias in the electronic health record. Health Affairs 41(2) [https://www.healthaffairs.org/doi/10.1377/hlthaff.2021.01423]
- Gervasi SS, Chen IY, Smith-McLallen A, Sontag D, Obermeyer Z, Vennera M, Chawla R (2022) The potential for bias in machine learning and opportunities for health insurers to address it. Health Affairs 41(2) [https://www.healthaffairs.org/doi/10.1377/hlthaff.2021.01287]
- Pierson E, Cutler DM, Leskovec J, Mullainathan S, Obermeyer Z (2021) An algorithmic approach to reducing unexplained pain disparities in underserved populations. Nature Medicine 27: 136-140. [https://www.nature.com/articles/s41591-020-01192-7]
- Amutah C, Greenidge K, Mante A, Munyikwa M, Surya SL, Higginbotham E, Jones DS, Lavizzo-Mourey R, Roberts D, Tsai J, Aysola J (2021) Misrepresenting race – the role of medical schools in propagating physician bias. The New England Journal of Medicine 384: 872-878 [https://www.nejm.org/doi/full/10.1056/nejmms2025768]
- Jillson E (2021) Aiming for truth, fairness and equity in your company’s use of AI. Federal Trade Commission Business Blog [https://www.ftc.gov/business-guidance/blog/2021/04/aiming-truth-fairness-equity-your-companys-use-ai]
- Raji ID, Smart A, White RN, Mitchell M, Gebru T, Hutchinson B, Smith-Loud J, Theron D, Barnes P (2020) Closing the AI accountability gap: defining end-to-end framework for internal algorithmic auditing. Accepted to ACM FAT* (Fariness, Accountability and Transparency) conference 2020 [https://arxiv.org/abs/2001.00973]
- Obermeyer Z, Powers B, Vogeli C, Mullainathan S (2019) Dissecting racial bias in an algorithm used to manage the health of populations. Science 366(6464): 447-453. [https://www.science.org/doi/10.1126/science.aax2342]
- Chen IY, Johansson FD, Sontag D (2018) Why is my classifier discriminatory? Advances in Neural Information Processing Systems 31: 3543-3554. [https://arxiv.org/abs/1805.12002]
- Buolamwini J, Gebru T (2018) Gender shades: intersectional accuracy disparities in commercial gender classification. Proceedings of Machine Learning Research 81: 1-15. [http://proceedings.mlr.press/v81/buolamwini18a/buolamwini18a.pdf]
- The Algorithmic Bias Playbook: A guide for C-suite leaders, technical teams, policymakers, and regulators on how to define, measure, and mitigate bias in live algorithms [https://www.chicagobooth.edu/research/center-for-applied-artificial-intelligence/research/algorithmic-bias/playbook]